The STAT News Report: A Wake-Up Call for AI Drug Discovery
As industries mature, moments like this reveal the true nature of companies—some double down on hype, while others step forward with data, proof-of-concept, and real impact. AI-driven drug discovery is at this inflection point.

It is no coincidence that the STAT News report and the Fierce Biotech transparency report were released in the same week. As industries mature, moments like this reveal the true nature of companies—some double down on hype, while others step forward with data, proof of concept, and real impact. AI-driven drug discovery is at this inflection point. The widening gap between exaggerated claims and measurable results has never been more apparent, making transparency the defining factor in separating innovation from marketing spin.
In the recent STAT News report, Brittany Trang put the spotlight on the AI-driven drug discovery industry, exposing how some biotech companies exaggerate their capabilities. The report revealed that companies like Absci and Generate Biomedicines claimed to be designing drugs from scratch with AI when, in reality, they were mostly tweaking existing molecules - “AI pharma and biotech companies’ breathless claims can potentially mislead anyone not intimately familiar with the details of drug development, or machine learning.” This has fueled skepticism across the biotech community, especially on Reddit forums, where industry insiders have voiced concerns about overhyped claims.
In contrast to the STAT News report, Conor Hale’s Fierce Biotech article highlighted the rapid advancements and promise of AI-driven drug discovery, emphasizing how AI can reduce timelines and expand chemical space. Unlike the STAT News critique of overhyped claims, Fierce Biotech focused on the potential of AI when properly applied, showcasing how companies with robust methodologies are leading the charge. This contrast underscores the divide between those leveraging AI effectively and those using it as a buzzword to attract investment.
Amidst the noise, companies are proving that AI drug discovery, when done right, is more than just hype. Insilico Medicine and Model Medicines show what real AI-driven drug development looks like—through transparency, leadership, and rigorous science.
Insilico Medicine: A Light in the Darkness
While some companies have been called out for vague claims, Insilico Medicine has taken a different approach—sharing real data. Their recent report provides concrete benchmarks, demonstrating:
An average 13-month timeline from initial discovery to a lead candidate (traditional methods take 2.5–4 years).
A 100% success rate for moving from preclinical candidate to IND-enabling studies (excluding voluntarily discontinued programs).
A clear look at their process, including how many molecules they tested before landing on a winner (~70 per program).
This kind of openness sets a new standard for the AI drug discovery industry. Instead of making grand claims without proof, Insilico is showing what AI can achieve through methodical, transparent research. Real breakthroughs don’t need exaggeration—they need data.
Model Medicines: Leading with Transparency and Science
At Model Medicines, we’ve always believed that results, not rhetoric, should measure AI-driven drug discovery. That’s why we developed the AIDD Code, a set of six key criteria that define success in AI-powered drug discovery:
New Biology & Target Discovery – Finding novel biological pathways, not just reusing old ones.
New Chemical Entities – Creating brand-new molecules, not minor modifications of existing drugs.
Zero-Shot Hit Rates >30% at <20μM – At least 30% of AI-predicted compounds must show real biological activity.
Tanimoto Scores <0.5 – Ensuring AI-generated molecules are genuinely new, not slight tweaks.
A Diverse Pipeline – Spanning multiple disease areas, proving AI’s broad application.
Preclinical Proof-of-Concept – Showing real efficacy in gold-standard animal models.
We don’t just talk about these criteria—we meet them and even exceed them. Our GALILEO™ platform recently achieved a 100% hit rate in our most recent preprint, proving that AI can drive real drug discovery success. By focusing on hard data instead of hype, we aim to build trust and credibility in this space.
Below are the preprints that demonstrate our platform, target discovery, drug discovery, and drug development capabilities:
The Power of Zero-Shot Hit Rates and Tanimoto Scores
One of the biggest takeaways from the STAT News report is the lack of real success metrics in AI drug discovery. Many companies claim their AI “discovers” drugs but fail to share the numbers behind their claims. We focus on key metrics like zero/one-shot hit rates and Tanimoto scores—because results matter.
Zero-Shot: A zero-shot prediction refers to an AI model’s ability to identify novel chemical entities (NCEs) with a desired biological activity when no known examples from the same molecular class are present in the training set.
One-Shot: A one-shot prediction refers to an AI model’s ability to identify novel chemical entities (NCEs) with a desired biological activity when the training set contains only a single known example from the same molecular class.
In our most recent preprint, we demonstrate:
100% of our one-shot AI-identified compounds show real biological activity at <20μM on the first experimental try.
We’ve validated 12 out of 12 AI-designed compounds in disease models.
For context, traditional drug discovery hit rates are significantly lower. AI—when done correctly—can dramatically accelerate the process. But without transparency, the industry risks losing credibility. That’s why we share our numbers, and why we challenge others to do the same.
Equally important, Tanimoto Scores measure how structurally similar a newly designed compound is to known molecules. Many so-called AI-generated drugs are just small modifications of existing molecules. At Model Medicines, we set a high bar—Tanimoto scores must be <0.5 (lower is better) to ensure true novelty. If AI is simply predicting known chemical space or making minor tweaks to existing drugs, it’s not discovering.
In our latest preprint study, our 12 novel antiviral compounds had an average Tanimoto similarity of 0.38 to MDL-001 and 0.13 to Beclabuvir, meaning they are highly dissimilar from existing antiviral drugs. This is critical because AI should be expanding chemical space, not repeating past discoveries.
By focusing on zero-shot and one-shot hit rates and Tanimoto scores, we ensure that AI-driven drug discovery is actually producing novel, active compounds—not just rehashed versions of existing drugs. To learn more, check out AI Hit Rates and Novelty.
The Future of AI Drug Discovery
Transparency as the Gold Standard
The STAT News report has drawn unprecedented attention to AI drug discovery, and it’s clear that this is a defining moment for the industry. Companies must decide whether to double down on vague marketing or embrace transparency and let the science speak for itself.
We at Model Medicines believe the answer is clear. Like Insilico Medicine, we are committed to sharing verifiable data, real hit rates, mathematical novelty, and tangible progress. This is how AI will truly revolutionize drug discovery—not through promises, but through results.
AI drug discovery doesn’t need hype. It needs leaders who prove their success through transparency, rigorous science, and real-world impact. The industry should follow suit.
Let’s raise the bar together.
Details
Date
Feb 19, 2025
Category
Insights
Reading
2 Mins
Author
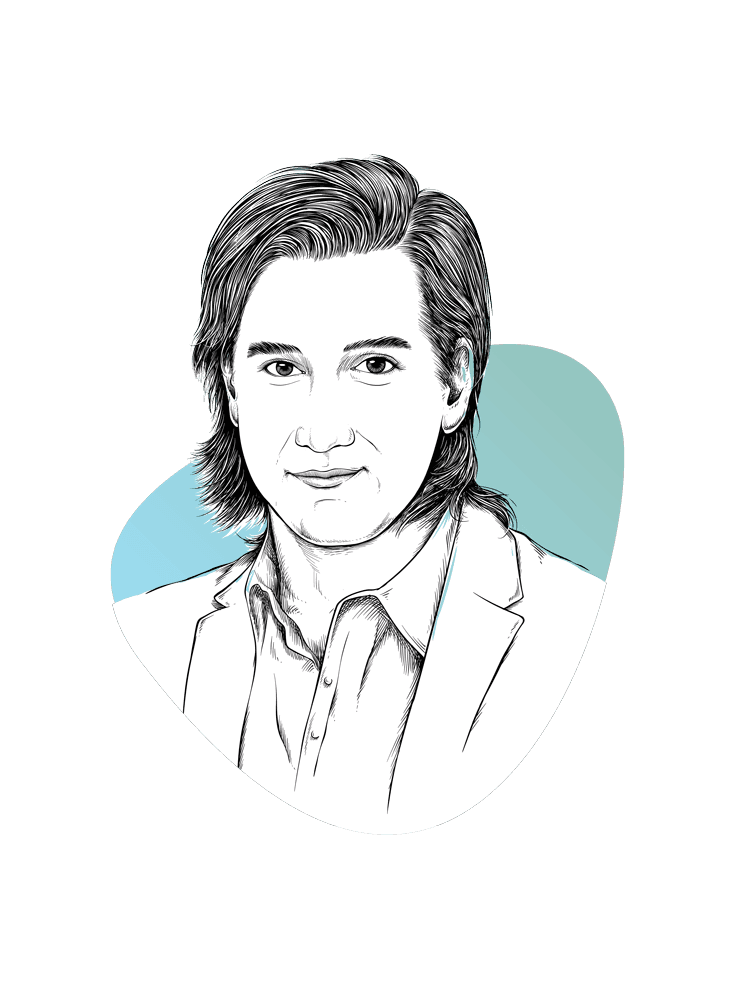
Patrick ONeill
Investor Relations
Related News