Vanity Metrics & Black Boxes ≠Therapeutics
Maximizing prospective model performance for AI drug discovery through field optimized data segmentation, adaptive molecular representations, novel subgraph explainability and rigorous validation.
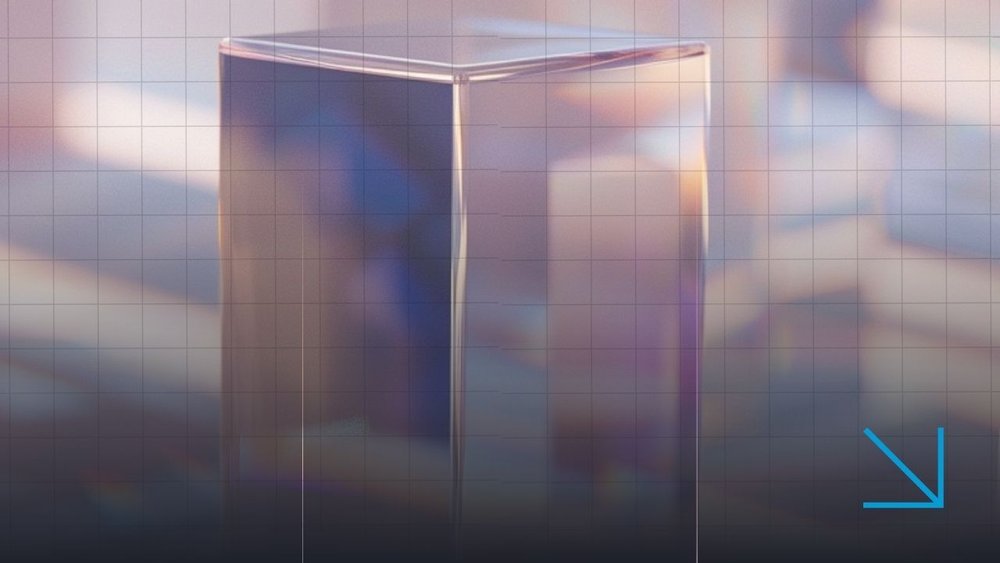
Maximizing prospective model performance for AI drug discovery through field optimized data segmentation, adaptive molecular representations, novel subgraph explainability and rigorous validation.
Model Medicines created and optimized its GALILEO™ AI Drug Discovery platform for prospective drug discovery, demonstrating a 45.5% discovery hit rate for cycle one of an oncology program and discovering MDL-001, a potential best-in-class therapeutic with preclinical animal model proof-of-concept.
This paper is part of a series that reviews various aspects of Model Medicines GALILEO™ AI Drug Discovery Platform and its two parallel drug discovery modules CHEMPrint™ and Constellation™ .
Each paper demonstrates the capabilities of the GALILEO™ platform through quantitative case studies. The first three CHEMPrint™ papers presented our proprietary, class-leading pharmacophore modeling data acquisition and curation pipeline [1], proprietary, class-leading AI-Graph Mining PAINS Classification model [2], and the rationale for the Target Product Profile (TPP) of our infectious disease program and MDL-001, a potential best-in-class therapeutic discovered using the CHEMPrint™ platform [3]. The first Constellation™ paper presented our proprietary, cutting edge Cryo-EM data acquisition pipeline [4].
Download
This paper focuses on the training, validation and performance of our Molecular Geometric Deep Learning (Mol-GDL)/Quantitative Structure Activity Relationship (QSAR) drug discovery production model, CHEMPrint™.
Details
Date
Sep 27, 2023
Category
Paper
Reading
2 Mins
Author
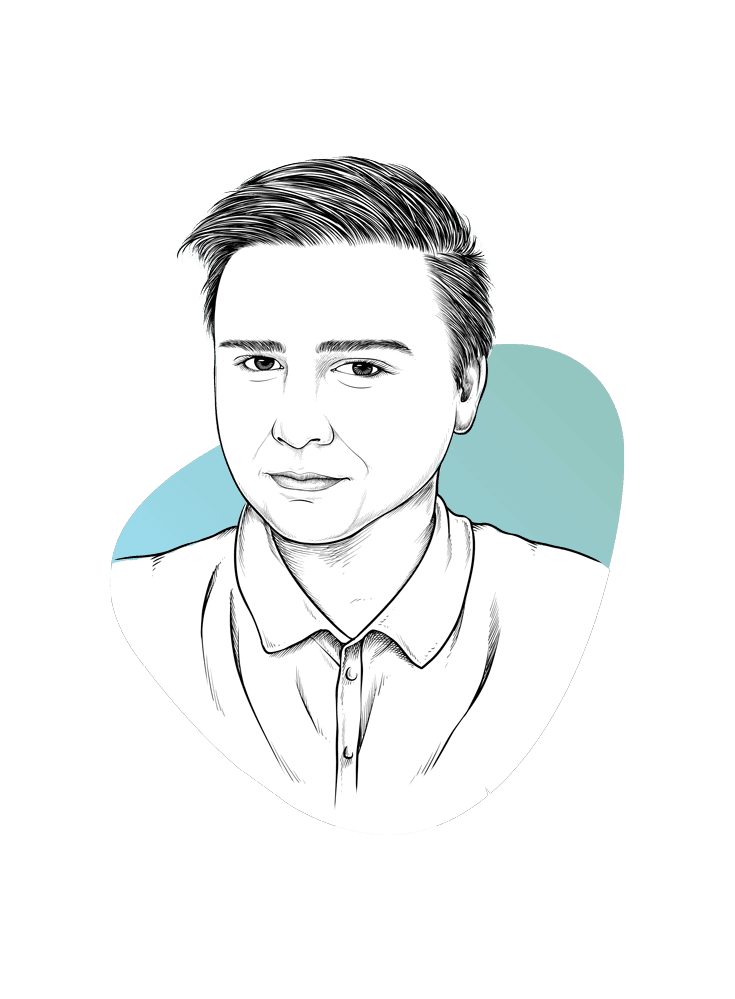
Virgil Woods
Fellow
Related News